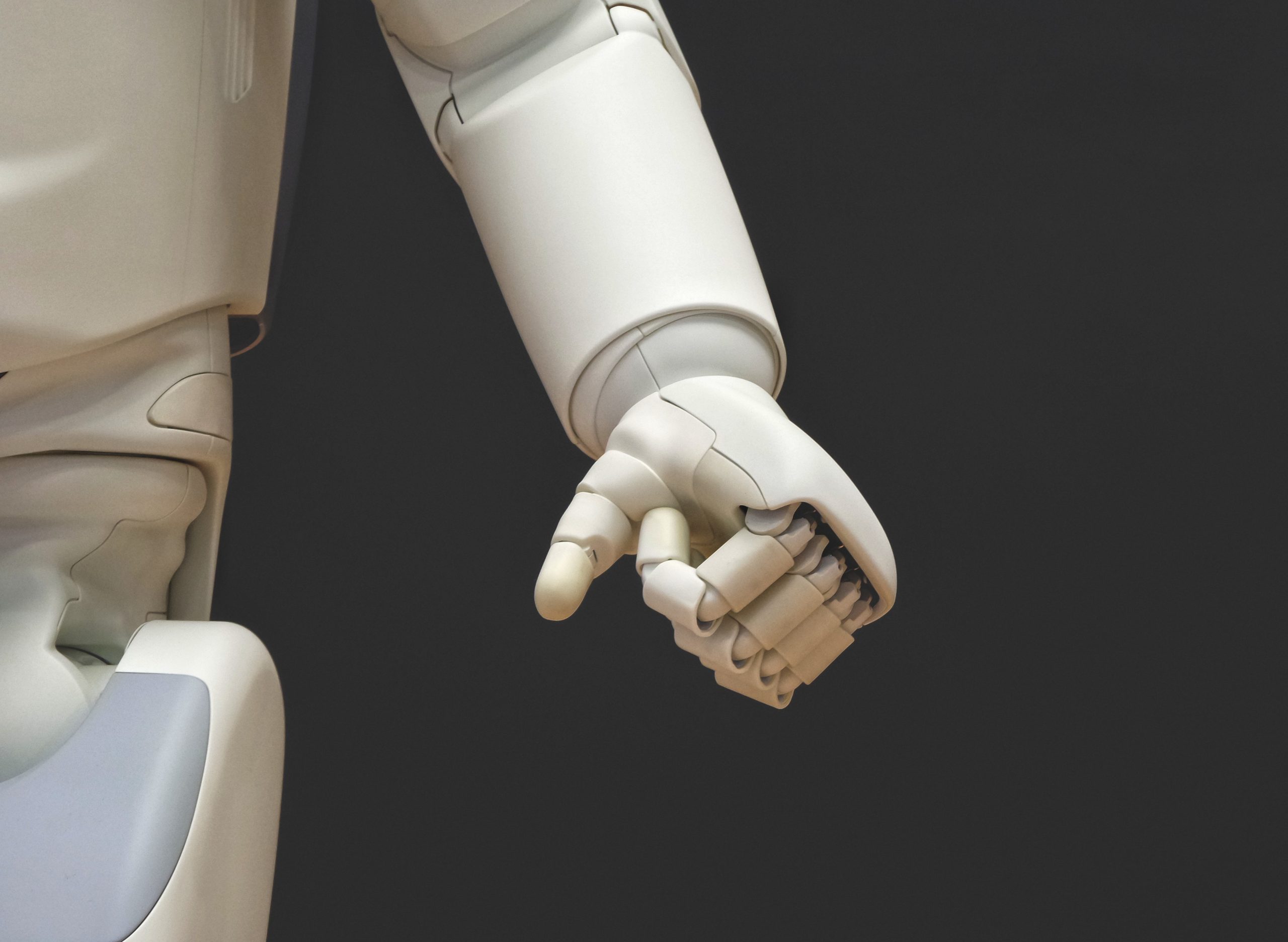
Can you imagine a sentient AI that has the same capabilities as us humans? Something that fills our imagination, the centerpiece of nearly all depictions of the far future, might be closer than ever. Or is it actually? What have scientists achieved so far, and do we have to be afraid of a Terminator-esque takeover?
Can you imagine a sentient AI that has the same capabilities as us humans? Something that fills our imagination, the centerpiece of nearly all depictions of the far future, might be closer than ever. Or is it actually? What have scientists achieved so far, and do we have to be afraid of a Terminator-esque takeover?
Photo by Possessed Photography
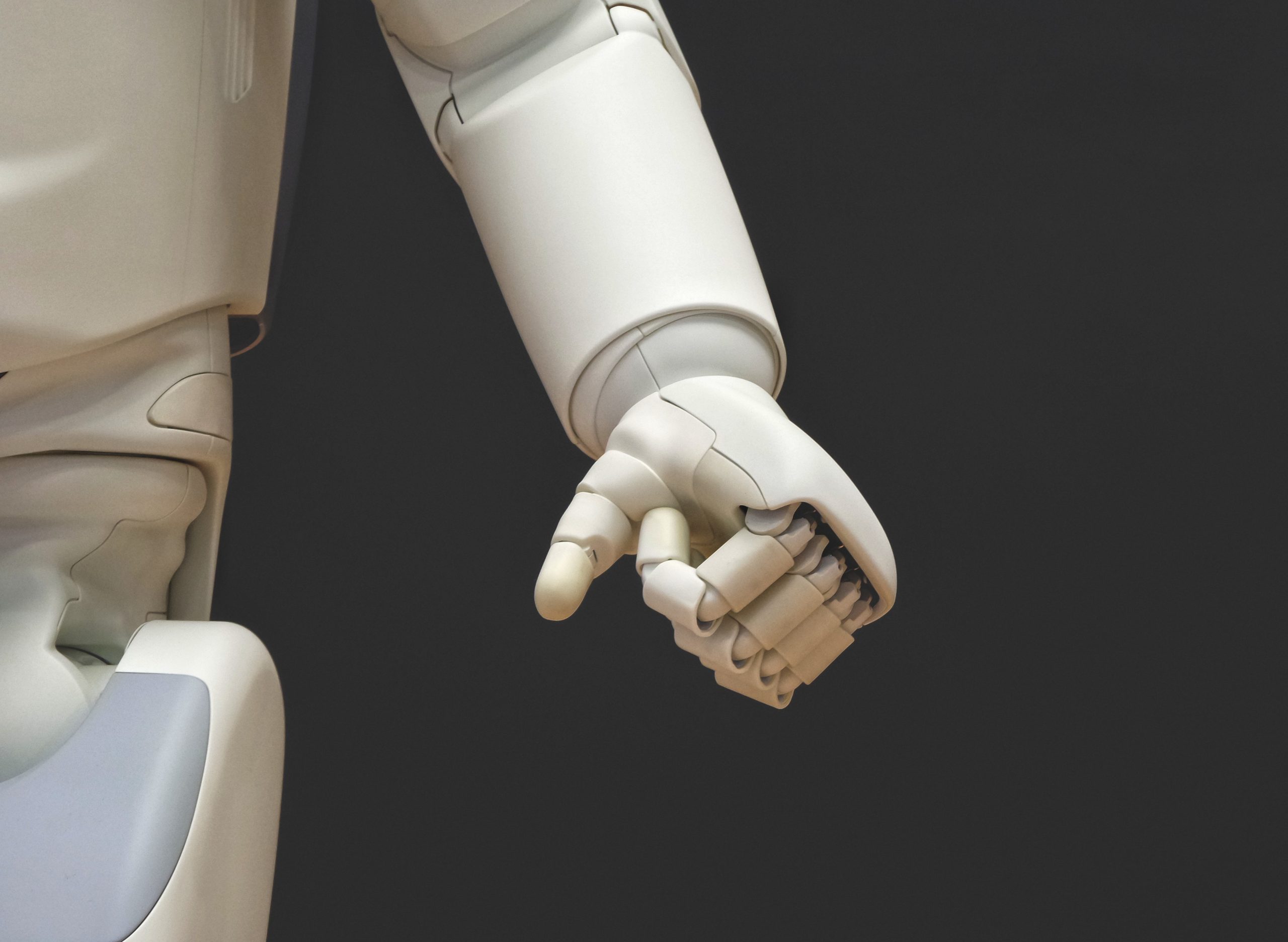
Photo by Possessed Photography
ChatGPT. Bing. Bixby. Siri. Google. These are all prime examples of artificial intelligence, programs that take inspiration from simulating human intelligence, showcasing how widespread the miscellaneous use of AI is in our lives (APA, 2023). From looking up recipes to debugging programming, everyone has a use for AI these days and it is not a far reach to say that this use has grown exponentially. But what happens in the background that makes them so intelligent? And can we really call it intelligence, or is it just pre-programmed computer smarts?
When considering ChatGPT, it seems like it is a mix of both. The developers of ChatGPT created a basic AI program that is taught by human AI trainers – people who are responsible for training AI to link prompts to answers. After multiple trials, these trainers are asked to rank the answers from best to worst, in order to develop a ‘reward model’. Once the AI picks up on how the rewards are being calculated, it can adjust its output for future prompts to best fit the reward model it has been trained with. Interestingly, it heavily resembles the reward circuit filled with dopamine inside our brains. It turns out, this is not the only biological mechanism that is being used in AI, and for the rest of the article I will be addressing how we are using biological mechanisms to better artificial intelligence, and whether it can be considered ‘alive’.
Being a predominant paradigm in AI development, biological mechanisms were in the spotlight long before the likes of ChatGPT. Narcross (2021) mentions that the three main methodologies of artificial intelligence – machine learning, representation and neuromorphic computing – all take inspiration from human biology. Machine learning is rooted in neural networks. One of its main components, Convolutional Neural Networks, extends this to being organized in the same fashion as neurons in the brain. Much like how neurons have varying computational power over each other, these nodes have variable weights in the network they belong, basing their connections to other nodes and the layers they reside in. Jeff Hawkins’ company Numenta also took this a step further by including temporal connectivity, the concept of time, in his modeling based on representation (Narcross, 2021). These methods, however, were largely limited to processing in a serial fashion, until a new paradigm called neuromorphic computing took the scene, with enough hardware to include parallel encoding, allowing AI systems to compute different solutions to the same prompt in parallel. As such, the inclusion of parallel encoding allowed modern AI to get a step closer to the human brain by replicating one of the key processes that occur during decision making. This allowed the capabilities of AI to expand even further. As such, we can easily say that biology is a big source of inspiration when it comes to AI development.
“ Maybe the problem is that we are not using the correct methods for a perfect replication; we are selfishly purely interested in the specific mechanisms that we are concerned about and not the brain as a whole.”
More and more scientists are trying to replicate biological processes in order to overcome hurdles in AI. Currently, we see scientists such as Poirazi (2023) researching the possible implications of introducing dendrites to AI modeling, to see if making the AI network more like a neural network will improve its processing. Moreover, Ji et al. (2021) managed to replicate associative learning mechanisms through an “ion-trapping non-volatile synaptic organic electrochemical transistor.” Simply put, they have designed a transistor machine that ‘associates’ light and pressure information for times as long as 200 minutes, compared to other associative learning mechanisms that rely on simultaneously firing electrical signals. As such, they are able to replicate processes in the brain not only in terms of the way neurons communicate, but in methods that we perceive as well, adding onto the link between biological processing and AI.
This also has benefits in advancing our knowledge about how our brain works. Simply put, if we can replicate certain processes in an artificial setting, we can manipulate as many variables as we like to discover more about the relationships between certain components in the brain. For example, for understanding how visual processing works, scientists have made AI models to decode fMRI input coming from V1 areas, to reconstruct what the brain perceives based on these recordings. Once the AI model gets according to correct matches of picture and V1 signal, it can be used to accurately decode the images one perceives in a dream (Miwayaki et al, 2008 and Horikawa et al., 2013, cited by Macpherson et al., 2021). This has led to the confirmation that perceived and imagined images use the same neural networks with similarly varied levels of visual areas, advancing our knowledge about how we process visual information. Even though this is not the only area that AI has advanced, we can clearly see that replicating biological processes with AI also reduces what we don’t know.
Introducing more biological mechanisms to AI to improve its processing and using it to understand more about our minds is an infinite loop. One question must be asked, however: can we ever perfectly replicate the brain in AI? Poirazi (2023) argues that we need to learn more about dendrites for her specific case, but the scientific community is straying further away from the methods used to measure dendritic activity because they are not ‘interesting’. Maybe the problem is that we are not using the correct methods for a perfect replication; we are selfishly purely interested in the specific mechanisms that we are concerned about and not the brain as a whole. So, should we try to aspire for a cohesive replication that aims for a one-to-one replication of the brain? The problem with this is that there are many types of components in our brain that we don’t know the specific function of, such as the types of interneurons. This leaves us with a dilemma. Should we try to replicate everything, which will take much more time and effort, or should we try to filter out what is necessary according to our own knowledge? This seems to be the question that prevents us from making a leap in advancement. Ultimately, I don’t think we need to worry about AI being sentient for a while. <<
References
-
American Psychological Association. (2023). APA Dictionary of Psychology. American Psychological Association. https://dictionary.apa.org/artificial-intelligence
-
Horikawa, T., Tamaki, M., Miyawaki, Y., & Kamitani, Y. (2013). Neural decoding of visual imagery during sleep. Science, 340(6132), 639–642. https://doi.org/10.1126/science.1234330
-
Ji, X., Paulsen, B. D., Chik, G. K., Wu, R., Yin, Y., Chan, P. K., & Rivnay, J. (2021). Mimicking associative learning using an ion-trapping non-volatile synaptic organic electrochemical transistor. Nature Communications, 12(1). https://doi.org/10.1038/s41467-021-22680-5
-
Kozachkov, L., Kastanenka, K. V., & Krotov, D. (2023). Building transformers from neurons and astrocytes. Proceedings of the National Academy of Sciences, 120(34). https://doi.org/10.1073/pnas.2219150120
-
Macpherson, T., Churchland, A., Sejnowski, T., DiCarlo, J., Kamitani, Y., Takahashi, H., & Hikida, T. (2021). Natural and artificial intelligence: A brief introduction to the interplay between AI and neuroscience research. Neural Networks, 144, 603–613. https://doi.org/10.1016/j.neunet.2021.09.018
-
Middlebrooks, P., & Poirazi, P. (2023). BI 167 Panayiota Poirazi: AI Brains Need Dendrites. Brain Inspired. other. Retrieved September 30, 2023,.
-
Narcross, F. (2021). Artificial Nervous Systems—a new paradigm for Artificial Intelligence. Patterns, 2(6), 100265. https://doi.org/10.1016/j.patter.2021.100265
-
OpenAI. (2022). Introducing chatgpt. Introducing ChatGPT. https://openai.com/blog/chatgpt
ChatGPT. Bing. Bixby. Siri. Google. These are all prime examples of artificial intelligence, programs that take inspiration from simulating human intelligence, showcasing how widespread the miscellaneous use of AI is in our lives (APA, 2023). From looking up recipes to debugging programming, everyone has a use for AI these days and it is not a far reach to say that this use has grown exponentially. But what happens in the background that makes them so intelligent? And can we really call it intelligence, or is it just pre-programmed computer smarts?
When considering ChatGPT, it seems like it is a mix of both. The developers of ChatGPT created a basic AI program that is taught by human AI trainers – people who are responsible for training AI to link prompts to answers. After multiple trials, these trainers are asked to rank the answers from best to worst, in order to develop a ‘reward model’. Once the AI picks up on how the rewards are being calculated, it can adjust its output for future prompts to best fit the reward model it has been trained with. Interestingly, it heavily resembles the reward circuit filled with dopamine inside our brains. It turns out, this is not the only biological mechanism that is being used in AI, and for the rest of the article I will be addressing how we are using biological mechanisms to better artificial intelligence, and whether it can be considered ‘alive’.
Being a predominant paradigm in AI development, biological mechanisms were in the spotlight long before the likes of ChatGPT. Narcross (2021) mentions that the three main methodologies of artificial intelligence – machine learning, representation and neuromorphic computing – all take inspiration from human biology. Machine learning is rooted in neural networks. One of its main components, Convolutional Neural Networks, extends this to being organized in the same fashion as neurons in the brain. Much like how neurons have varying computational power over each other, these nodes have variable weights in the network they belong, basing their connections to other nodes and the layers they reside in. Jeff Hawkins’ company Numenta also took this a step further by including temporal connectivity, the concept of time, in his modeling based on representation (Narcross, 2021). These methods, however, were largely limited to processing in a serial fashion, until a new paradigm called neuromorphic computing took the scene, with enough hardware to include parallel encoding, allowing AI systems to compute different solutions to the same prompt in parallel. As such, the inclusion of parallel encoding allowed modern AI to get a step closer to the human brain by replicating one of the key processes that occur during decision making. This allowed the capabilities of AI to expand even further. As such, we can easily say that biology is a big source of inspiration when it comes to AI development.
“ Maybe the problem is that we are not using the correct methods for a perfect replication; we are selfishly purely interested in the specific mechanisms that we are concerned about and not the brain as a whole.”
More and more scientists are trying to replicate biological processes in order to overcome hurdles in AI. Currently, we see scientists such as Poirazi (2023) researching the possible implications of introducing dendrites to AI modeling, to see if making the AI network more like a neural network will improve its processing. Moreover, Ji et al. (2021) managed to replicate associative learning mechanisms through an “ion-trapping non-volatile synaptic organic electrochemical transistor.” Simply put, they have designed a transistor machine that ‘associates’ light and pressure information for times as long as 200 minutes, compared to other associative learning mechanisms that rely on simultaneously firing electrical signals. As such, they are able to replicate processes in the brain not only in terms of the way neurons communicate, but in methods that we perceive as well, adding onto the link between biological processing and AI.
This also has benefits in advancing our knowledge about how our brain works. Simply put, if we can replicate certain processes in an artificial setting, we can manipulate as many variables as we like to discover more about the relationships between certain components in the brain. For example, for understanding how visual processing works, scientists have made AI models to decode fMRI input coming from V1 areas, to reconstruct what the brain perceives based on these recordings. Once the AI model gets according to correct matches of picture and V1 signal, it can be used to accurately decode the images one perceives in a dream (Miwayaki et al, 2008 and Horikawa et al., 2013, cited by Macpherson et al., 2021). This has led to the confirmation that perceived and imagined images use the same neural networks with similarly varied levels of visual areas, advancing our knowledge about how we process visual information. Even though this is not the only area that AI has advanced, we can clearly see that replicating biological processes with AI also reduces what we don’t know.
Introducing more biological mechanisms to AI to improve its processing and using it to understand more about our minds is an infinite loop. One question must be asked, however: can we ever perfectly replicate the brain in AI? Poirazi (2023) argues that we need to learn more about dendrites for her specific case, but the scientific community is straying further away from the methods used to measure dendritic activity because they are not ‘interesting’. Maybe the problem is that we are not using the correct methods for a perfect replication; we are selfishly purely interested in the specific mechanisms that we are concerned about and not the brain as a whole. So, should we try to aspire for a cohesive replication that aims for a one-to-one replication of the brain? The problem with this is that there are many types of components in our brain that we don’t know the specific function of, such as the types of interneurons. This leaves us with a dilemma. Should we try to replicate everything, which will take much more time and effort, or should we try to filter out what is necessary according to our own knowledge? This seems to be the question that prevents us from making a leap in advancement. Ultimately, I don’t think we need to worry about AI being sentient for a while. <<
References
-
American Psychological Association. (2023). APA Dictionary of Psychology. American Psychological Association. https://dictionary.apa.org/artificial-intelligence
-
Horikawa, T., Tamaki, M., Miyawaki, Y., & Kamitani, Y. (2013). Neural decoding of visual imagery during sleep. Science, 340(6132), 639–642. https://doi.org/10.1126/science.1234330
-
Ji, X., Paulsen, B. D., Chik, G. K., Wu, R., Yin, Y., Chan, P. K., & Rivnay, J. (2021). Mimicking associative learning using an ion-trapping non-volatile synaptic organic electrochemical transistor. Nature Communications, 12(1). https://doi.org/10.1038/s41467-021-22680-5
-
Kozachkov, L., Kastanenka, K. V., & Krotov, D. (2023). Building transformers from neurons and astrocytes. Proceedings of the National Academy of Sciences, 120(34). https://doi.org/10.1073/pnas.2219150120
-
Macpherson, T., Churchland, A., Sejnowski, T., DiCarlo, J., Kamitani, Y., Takahashi, H., & Hikida, T. (2021). Natural and artificial intelligence: A brief introduction to the interplay between AI and neuroscience research. Neural Networks, 144, 603–613. https://doi.org/10.1016/j.neunet.2021.09.018
-
Middlebrooks, P., & Poirazi, P. (2023). BI 167 Panayiota Poirazi: AI Brains Need Dendrites. Brain Inspired. other. Retrieved September 30, 2023,.
-
Narcross, F. (2021). Artificial Nervous Systems—a new paradigm for Artificial Intelligence. Patterns, 2(6), 100265. https://doi.org/10.1016/j.patter.2021.100265
-
OpenAI. (2022). Introducing chatgpt. Introducing ChatGPT. https://openai.com/blog/chatgpt