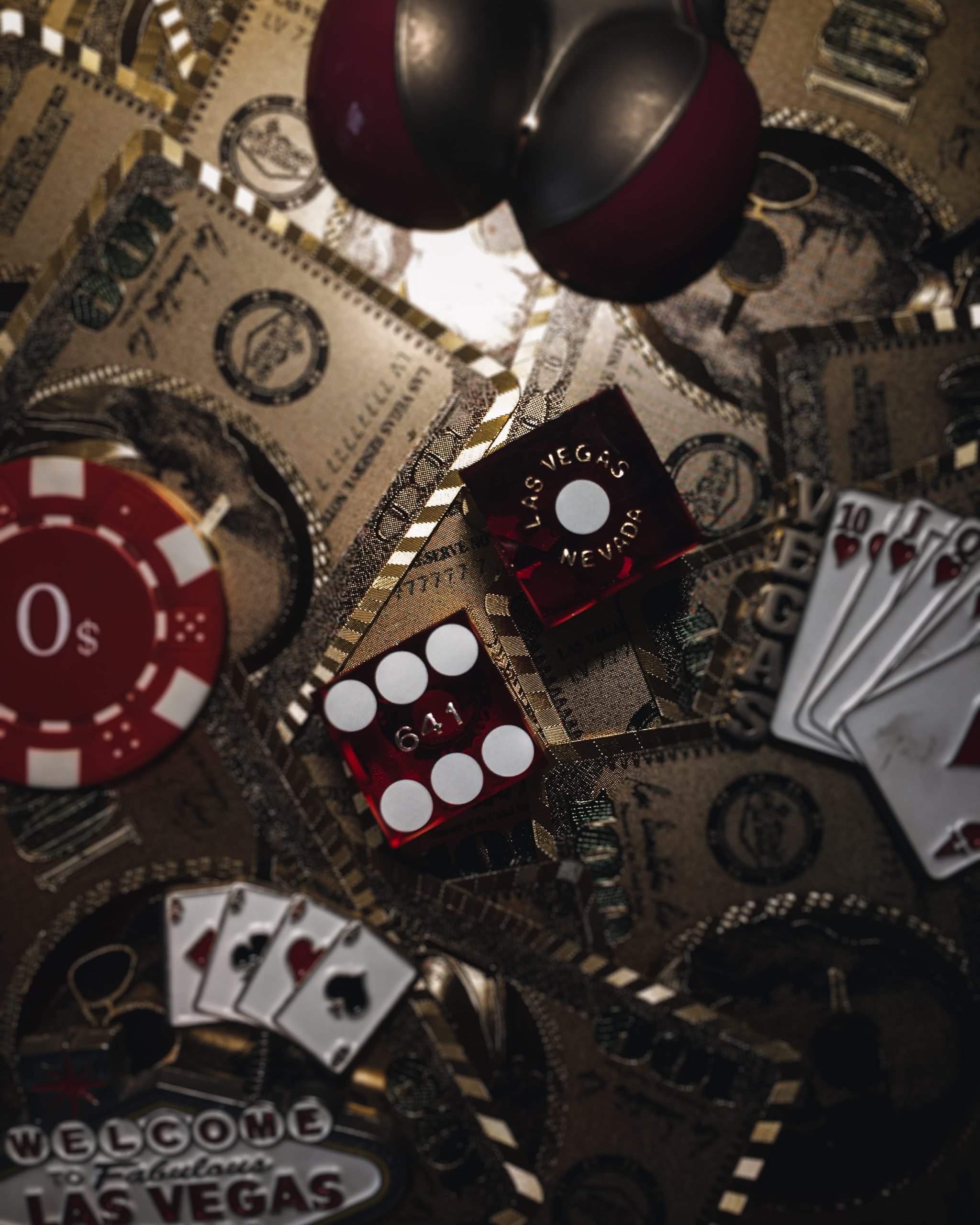
Should you search for a new movie to watch or enjoy an old favourite? Try a different type of coffee or get your usual? Should we fund clinical trials of new and emerging treatments or stick to the tried-and-tested ones? Where to invest? Where to drill for oil? Where to get tonight’s dinner from? In other words, should you explore or exploit?
Should you search for a new movie to watch or enjoy an old favourite? Try a different type of coffee or get your usual? Should we fund clinical trials of new and emerging treatments or stick to the tried-and-tested ones? Where to invest? Where to drill for oil? Where to get tonight’s dinner from? In other words, should you explore or exploit?
When we hear the word ‘exploration’, we think of flexibility, chance, play, experimentation, risk, search, innovation, adventure. Exploration is simply the gathering of new information. As for ‘exploitation’, it’s about using information you already have to achieve a known good result. It implies efficiency, safety, control, reliability and standardisation.
Together they comprise a dilemma – the allure of the unknown? Or the comfort of the familiar?
It is easy to love a favourite book, old friends, or the greatest hit at a concert. But the dilemma arises precisely because ‘the unknown’ is, well, unknown. It is a gamble: it could be a future favourite, the worst discovery ever, or anywhere in between. As author Brian Christian, who researches decision-making, points out, every ‘best’ began humbly as something ‘new’ (Christian & Griffiths, 2016) – a reminder that undiscovered future favourites are out there.
Speaking of gambles, a hypothetical casino can help us understand the explore-exploit dilemma, also known as ‘the multi-armed bandit problem’. A ‘one-armed bandit’ is simply one slot machine with one lever. If you pull the lever, you might get a cash reward. Each machine has its own probability distribution of giving that reward. A row of many such machines forms the ‘multi-armed bandit’. At first, you don’t know the payout rate of any machine. And as you start pulling a few levers, it becomes clear that some machines are more rewarding than others. Ultimately, you want to maximise your rewards. That’s where the dilemma kicks in – as the night progresses, should you secure some reward by ‘exploiting’ the machines that seem promising so far? Or should you explore different machines to perhaps discover one that’s much more rewarding than the machines you’re currently playing?
We can simplify this question: imagine you enter a casino and there are two slot machines. You pull the first one 15 times, and it pays off 9 times. Then you move to the other machine, and you pull it twice, it pays off once. Now, you need to evaluate the machines. Don’t start multiplying for an expected value just yet; because the question isn’t ‘which arm should you pull next?’ You need to consider more than just the next pull because there are very many pulls to come – the more important question is: ‘how long will you stay at the casino?’
Strategy 1 – Time Interval
So, why is there a difference between the next pull and all the future pulls of the night? Data scientist Chris Stucchio’s analogy can help us understand: “I’m more likely to try a new restaurant when I move to a city than when I’m leaving it,” he says, “and as I leave the city, I go back to all my old favourites rather than trying out new stuff.” He chooses to explore if he’s going to stick around for a while, and to exploit if he knows that he’s leaving soon. This is not a lesson in loyalty, but a lesson in decision-making. “Because even if I did find a slightly better restaurant [as I’m leaving], I’m only going to go there once or twice, so why take the risk?” According to him, the interval of time you have to explore or exploit makes all the difference.
To see the importance of the time interval, we can ask ourselves – what is the point of exploration? We explore because we want to discover which options are good, so that in the future we can exploit those options. If casino night ends in 15 minutes, don’t risk exploring new machines. Because even if you find slightly better odds (and you might not), you don’t have time to exploit them. But if the night has just begun, then you’ve got all the time in the world to find your jackpot. The ultimate lesson is to decrease exploration over time, because the opportunities and time left to savour your finds reduce; and to increase exploitation over time, that is, to cash in. However, we often don’t know how long the interval will be. For example, I don’t know how long I’ll stay in Amsterdam, so should I explore new cafes, or exploit my finds?
Strategy 2 – Regret Minimisation
One strategy is to think about regret. Regret is usually our biggest fear when making risky decisions. It isn’t about the choices we have made but rather the ones we could have made, but did not. How can we factor in regret into our explore-exploit balance?
Once upon a time, a man left a nice and safe investment job in NYC to sell books online, even though his boss had advised him not to. Many years later, that online bookstore became Amazon.com. In an interview, Jeff Bezos talked about how he made the decision to take the leap: “I knew that if I failed I wouldn’t regret that, but I knew the one thing I might regret is not ever having tried…when I thought about it that way it was an incredibly easy decision.”
Here, Bezos followed what he called ‘regret minimisation’. His priority was to avoid future disappointment, not to find the objectively best option. We can apply regret minimisation to our casino night. Instead of thinking “which machine performed the best so far?”, think of “Which one could perform the best in the future?” Regret minimization is about looking beyond past performance and focusing on future potential. It’s about looking at so-called ‘upper confidence bounds’ which simply means the ‘potential reward’ that you could take home. When you have options to explore, there is always risk and uncertainty, but you should give all options the benefit of doubt and expect from them the best they can offer.
This strategy hinges on ‘optimism in the face of uncertainty’. We don’t care about the lower bound – we aren’t looking at the worst case scenario. Instead we put a smile on our face, think positive thoughts, and choose whatever has the most potential. A new cafe could be amazing, and going there once or twice may not be enough information to decide whether it’s better or worse than your favourites. Just like in research, when you don’t have many observations, the confidence interval remains wide. It’s possible that the cafe could prove itself to be the best. The more you visit it, the more information and experience you get, the narrower the confidence interval gets, the better you can decide. By being optimistic about the cafe, you give it a chance to amaze you. Similarly, in clinical trials, being optimistic about initial studies and giving new-and-emerging treatments a fair empirical chance, we open ourselves up to the possibility of discovering a groundbreaking solution.
Whether they’re about cafes or about clinical trials, decisions aren’t one-time gambles. They’re a series of pulls, with varying potential, uncertain intervals, unknown risks and rewards: the exploration-exploitation tradeoff is a journey. Intervals and optimism can be the compass. <<
References
-
Rhee, M., & Kim, T. (2018). Exploration and exploitation. In Palgrave Macmillan UK eBooks (pp. 543–546). https://doi.org/10.1057/978-1-137-00772-8_388
-
Christian, B. R., & Griffiths, T. (2016). Algorithms to Live By: The Computer Science of Human Decisions. https://openlibrary.org/books/OL25935646M/Algorithms_to_live_by
-
Jeff Bezos, Academy Class of 2001, Part 12. (n.d.). Academy of Achievement. Retrieved October 17, 2023, from https://achievement.org/video/jeff-bezos-12/
-
GeeksforGeeks. (2020, February 19). Upper Confidence bound Algorithm in Reinforcement learning. https://www.geeksforgeeks.org/upper-confidence-bound-algorithm-in-reinforcement-learning/
When we hear the word ‘exploration’, we think of flexibility, chance, play, experimentation, risk, search, innovation, adventure. Exploration is simply the gathering of new information. As for ‘exploitation’, it’s about using information you already have to achieve a known good result. It implies efficiency, safety, control, reliability and standardisation.
Together they comprise a dilemma – the allure of the unknown? Or the comfort of the familiar?
It is easy to love a favourite book, old friends, or the greatest hit at a concert. But the dilemma arises precisely because ‘the unknown’ is, well, unknown. It is a gamble: it could be a future favourite, the worst discovery ever, or anywhere in between. As author Brian Christian, who researches decision-making, points out, every ‘best’ began humbly as something ‘new’ (Christian & Griffiths, 2016) – a reminder that undiscovered future favourites are out there.
Speaking of gambles, a hypothetical casino can help us understand the explore-exploit dilemma, also known as ‘the multi-armed bandit problem’. A ‘one-armed bandit’ is simply one slot machine with one lever. If you pull the lever, you might get a cash reward. Each machine has its own probability distribution of giving that reward. A row of many such machines forms the ‘multi-armed bandit’. At first, you don’t know the payout rate of any machine. And as you start pulling a few levers, it becomes clear that some machines are more rewarding than others. Ultimately, you want to maximise your rewards. That’s where the dilemma kicks in – as the night progresses, should you secure some reward by ‘exploiting’ the machines that seem promising so far? Or should you explore different machines to perhaps discover one that’s much more rewarding than the machines you’re currently playing?
We can simplify this question: imagine you enter a casino and there are two slot machines. You pull the first one 15 times, and it pays off 9 times. Then you move to the other machine, and you pull it twice, it pays off once. Now, you need to evaluate the machines. Don’t start multiplying for an expected value just yet; because the question isn’t ‘which arm should you pull next?’ You need to consider more than just the next pull because there are very many pulls to come – the more important question is: ‘how long will you stay at the casino?’
Strategy 1 – Time Interval
So, why is there a difference between the next pull and all the future pulls of the night? Data scientist Chris Stucchio’s analogy can help us understand: “I’m more likely to try a new restaurant when I move to a city than when I’m leaving it,” he says, “and as I leave the city, I go back to all my old favourites rather than trying out new stuff.” He chooses to explore if he’s going to stick around for a while, and to exploit if he knows that he’s leaving soon. This is not a lesson in loyalty, but a lesson in decision-making. “Because even if I did find a slightly better restaurant [as I’m leaving], I’m only going to go there once or twice, so why take the risk?” According to him, the interval of time you have to explore or exploit makes all the difference.
To see the importance of the time interval, we can ask ourselves – what is the point of exploration? We explore because we want to discover which options are good, so that in the future we can exploit those options. If casino night ends in 15 minutes, don’t risk exploring new machines. Because even if you find slightly better odds (and you might not), you don’t have time to exploit them. But if the night has just begun, then you’ve got all the time in the world to find your jackpot. The ultimate lesson is to decrease exploration over time, because the opportunities and time left to savour your finds reduce; and to increase exploitation over time, that is, to cash in. However, we often don’t know how long the interval will be. For example, I don’t know how long I’ll stay in Amsterdam, so should I explore new cafes, or exploit my finds?
Strategy 2 – Regret Minimisation
One strategy is to think about regret. Regret is usually our biggest fear when making risky decisions. It isn’t about the choices we have made but rather the ones we could have made, but did not. How can we factor in regret into our explore-exploit balance?
Once upon a time, a man left a nice and safe investment job in NYC to sell books online, even though his boss had advised him not to. Many years later, that online bookstore became Amazon.com. In an interview, Jeff Bezos talked about how he made the decision to take the leap: “I knew that if I failed I wouldn’t regret that, but I knew the one thing I might regret is not ever having tried…when I thought about it that way it was an incredibly easy decision.”
Here, Bezos followed what he called ‘regret minimisation’. His priority was to avoid future disappointment, not to find the objectively best option. We can apply regret minimisation to our casino night. Instead of thinking “which machine performed the best so far?”, think of “Which one could perform the best in the future?” Regret minimization is about looking beyond past performance and focusing on future potential. It’s about looking at so-called ‘upper confidence bounds’ which simply means the ‘potential reward’ that you could take home. When you have options to explore, there is always risk and uncertainty, but you should give all options the benefit of doubt and expect from them the best they can offer.
This strategy hinges on ‘optimism in the face of uncertainty’. We don’t care about the lower bound – we aren’t looking at the worst case scenario. Instead we put a smile on our face, think positive thoughts, and choose whatever has the most potential. A new cafe could be amazing, and going there once or twice may not be enough information to decide whether it’s better or worse than your favourites. Just like in research, when you don’t have many observations, the confidence interval remains wide. It’s possible that the cafe could prove itself to be the best. The more you visit it, the more information and experience you get, the narrower the confidence interval gets, the better you can decide. By being optimistic about the cafe, you give it a chance to amaze you. Similarly, in clinical trials, being optimistic about initial studies and giving new-and-emerging treatments a fair empirical chance, we open ourselves up to the possibility of discovering a groundbreaking solution.
Whether they’re about cafes or about clinical trials, decisions aren’t one-time gambles. They’re a series of pulls, with varying potential, uncertain intervals, unknown risks and rewards: the exploration-exploitation tradeoff is a journey. Intervals and optimism can be the compass. <<
References
-
Rhee, M., & Kim, T. (2018). Exploration and exploitation. In Palgrave Macmillan UK eBooks (pp. 543–546). https://doi.org/10.1057/978-1-137-00772-8_388
-
Christian, B. R., & Griffiths, T. (2016). Algorithms to Live By: The Computer Science of Human Decisions. https://openlibrary.org/books/OL25935646M/Algorithms_to_live_by
-
Jeff Bezos, Academy Class of 2001, Part 12. (n.d.). Academy of Achievement. Retrieved October 17, 2023, from https://achievement.org/video/jeff-bezos-12/
-
GeeksforGeeks. (2020, February 19). Upper Confidence bound Algorithm in Reinforcement learning. https://www.geeksforgeeks.org/upper-confidence-bound-algorithm-in-reinforcement-learning/